The term Industry 4.0 (I4.0) has been coined in Germany in 2011 at Hannover MESSE by Henning Kagermann and colleagues during the presentation of the German federal government’s high-tech strategy. In this latter occasion, they widespread the possibility of integration among information, communication technology as well as industrial processes. The strategy has been immediately seen with particular interest by the German government and the entire German manufacturing industry. The aforementioned proposed strategy aimed at strengthening and enhancing the competitiveness of industry and Kagermann and coworkers understood that the gap filling between ICT development and manufacturing world would have been the master key in order to pave the future way of German industry [1]. Since then, officially started the so-called fourth industrial revolution, also known as the Introduction of the Internet of Things into the production system [2], whose objective was to make the speed and cost of individual products produced by Small-Medium Enterprises (SMEs) comparable to those of mass production [3]. In this regard, a pilot study from Germany has evidenced how the companies clusters seemed to foster I4.0 development by facilitating the uptake and testing of I4.0 specific solutions [4].
However, it would be an oversimplification to state that the fourth industrial revolution is limited exclusively to the production process. The needed far-reaching change should also involve the value chain process from research and development to services concerning the final use of products, by exploiting and customizing the digital potential at different degrees in every single step along the value chain with a holistic approach [5]. Concerning the call for holistic path in the digitalization era, there are reported attempts in the literature where it has been tried to outline relevant descriptors for the “holistic management” of the productivity [6], as well as attempts to design strategy-oriented digitalization which enable an holistic view [7]. Starting from this work, in order to support senior managers and developers, Schallmo et al. have proposed a meta-view of holistic digitalization obtained by combining different fundamental perspective, such as: digital strategy, digital transformation, digital maturity and digital implementations [8]. As a matter of fact, the digital revolution has quickly found fertile implantation ground beyond the manufacturing process, being particularly suitable for the development of other sector, like the financial one for instance [9]. In addition, the intrinsic nature of lean manufacturing advocates for an holistic digital approach across the entire value chain and, in this regard, Valamede et al. have studied the integration potential among processes, digital technologies and stakeholders via three-step holistic methodology to drawn synergy points among I4.0 and Lean Manufacturing [10].
A synergistic cycling relation between humans, products and machines needs to be established along the value chain of manufacturing companies [11]. In order to achieve this goal, an infrastructure able to meet the requirements of the ever-increasing digitalization is needed, where both the enterprises and workers can benefit from the deriving increase in business competitiveness [12], [13] and from new flattened organizational hierarchies [14] To this latter extent, investments which finance the broadband network expansion and provide suitable technologies constitute a fundamental step. This expansion must be financed first by the private sector itself and, when the projects are not economically viable, companies must be aided by public subsidies [15].
Big data aspects should also not be neglected into the digital transformation, indeed they enable a significant decrease in business uncertainty, since information related to the business environment can be processed and analysed in almost real time and then passed directly to production facilities [16]. Furthermore, big data handling (like cloud computing et cetera) allows for a decentralization of the production system [17], by enabling a decentralization of the information which is located directly within the product itself, rather than centrally stored. This process further affects the decision-making system [18]. Based on the above mentioned criteria, the fourth industrial revolution can be defined as a systemic increase in the flexibility and customization of products and processes while retaining speed and efficiency, through automation, extensive network and decentralized control.
In addition to the digitalization degree, a concept study has outlined that it would be advisable to introduce also the automation one as criterion, since, due to a lack of standards and methodology, it is rather challenging to assess the digitalization level of processes as well as the automation degree facilitates conclusions drawing about the digitalization one, especially in the area of supporting activities. Starting from these considerations, Eva Bogner et al. developed a concept study based on two indices to analyze and report the status quo of the digitalization degree in addition to the automation one in the German manufacturing industry [5]. The first one corresponds to the level of digitalization across the entire value chain, while the second one determines the level of automation in production. As evidenced by the analyses of the surveyed companies in Germany, only one quarter of them will be able to withstand the disruptive changes of the fourth industrial revolution.
Germany has been a pioneer in recognizing and embracing the technological manufacturing trends as development gear [1], [19]. Therefore, initially conceived as a marketing tool, I4.0 has become a symbol of country determination to envisage its future as manufacturing worldwide major player. In terms of policies, the German Ministry of Education and Research has issued the first piece of legislation of the high-tech strategy in 2006 named as “Hightech Strategie”, which has been drafted by the joint efforts between the Forschungsunion (Development union) and the Expertenkommision Forschung und Innovation (Expert commission Research and Innovation) [20]. The objective of the cross-ministerial aforementioned strategy was to strengthen and to ensure a leading position in research and innovation for the country, as well as global production hub. The “Hightech Strategie” has implemented several cross-cutting activities and tools to achieve its goal, such as: increasing the linkages between the research sector and the private one, improvement of conditions for small medium enterprises and start-ups, investing in share and human capital and catalysing the diffusion of cutting-edge technologies [21].
Later on, in November 2010, Germany has launched the first holistic digital strategy, named “Deutschland Digital 2015” [22], where the way for a close collaboration among policy, business and research has been paved. Thanks to this business-oriented strategy, the potential of ICT industry (Information and Communications Technology) has been combined with the ones of more established industries, such as mechanical engineering or automobile industry, to achieve an intelligent network. Other strategies, discussed more in detail in the herein paper in section “Italian I4.0 scenario on policy level and cross-comparison with the German system” has then been introduced, until September 2018, when the latest strategy, titled “High-tech Strategy 2025- Research and Innovation that benefit the people” has been published [23]. This strategy has constituted a real breakthrough for the digital Industry, transitioning this latter one into the so-called 5.0 era [24], [25], whose origins can indeed be tracked back to Germany, where in Hannover in 2017 Japan presented a ground-breaking vision human-centred digital manufacturing vision [26].
While, although Italy represents the third largest EU economy, it outperforms as 18th out of 27 on the Digital Economy and Society Index (DESI). Nevertheless, the Italian score on DESI ranking over the past five years has advanced at remarkable pace and the gaps with the other EU countries need to be fulfilled in the near future in order to pave the crucial way which will enable EU as a whole to reach the 2030 Digital targets [27]. The herein presented paper aims at reviewing and outlining the current industrial digitalization scenario within the Italian system compared to the European one, the German in particular, as well as analysing the intrinsic systemic key-elements, which are responsible for the differentiated development [28], [29]. A particular attention is preserved for some of the most cutting-edge applications of AI for the industrial development, such as AI in research & development for accelerating material/product discovery through the identification of key-property-functions relations and also through predicting power. In this framework, its employment for catalyzing material discovery [30], [31] has apported a significant beneficial effect in various strategic field. For instance, AI and ML techniques became key-tools in the design process of mechanical materials [32], as well as in the energy-related field. In this latter regard, Tabor et al. discuss how an integrated artificial intelligence approach could potentially act as a game changer towards autonomous material discovery for clean energy [33]. Moreover, in more recent field of material research, such as electrocatalysis for sustainable chemical processes through the exploitation of renewable energy sources, ML has proven to be a promising tool for supporting the design process of electrocatalysts for CO2 reduction to chemical fuels [34], as well as for hydrogen and oxygen evolution reaction, oxygen reduction and nitrogen reduction [35]. In this context, AI has even proven to be capable to withstand with multiscale phenomena [36] and with the complex interplay of functionality and reactivity of 2D materials [37]. Moreover, in the pharmaceutical sector [38] and in the field of drug discovery, ML and AI constitute pivotal technologies for decreasing the number of measurements for each step typically performed in the traditional drug discovery process. This latter generally entails target identification and validation, assay development, high-throughput screening, lead optimization and pre-clinical trials. The reduced number of measurements and trials could in turn potentially reduce complexity and costs by saving raw materials, resources and energy [39]. In addition, the intrinsic potential of AI applications lies in its predictive power. Indeed ML-based supervised methods are capable to predict the outputs based on input features and this characteristic results particularly convenient in the case of precision medicine [40]. Among the folding fan of drugs, whose discovery has been accelerated by AI techniques, antibiotics play a major role. In this regard, deep learning techniques have revealed to be efficient in antibiotics discovery [41] and AI has even distinguished itself for its capability of aid in the design of small peptide biotics affective against a broad spectrum of superbugs [42].
On policylevel Italy has introduced the I4.0 dimension with a delay of ten years compared to the country which acted as the cradle of this process, such as Germany [20]. Indeed, the first legislative introduction of I4.0 National Plan (Piano Nazionale Industria 4.0) has been issued on September 2016 by Ministero dello Sviluppo Economico (Ministry level Italy has introduced the I4.0 dimension with a delay of ten years compared to the country which acted as the cradle of this process, such as Germany [20]. Indeed of Economic Development) [43]. The core of the plan was composed by the hyper- and super-amortization measures aimed at fiscally incentivizing companies which implemented cutting-edge technologies addressed to the I4.0 dimension of production processes. The plan included also other kind of financial benefits for companies, such as incentives tailored for innovation and R&D measures, involving start-ups and patents publications. As the “Hightech Strategie” and “Deutschland Digital 2015” launched in Germany in 2006 and 2010, respectively [20], [22], the Italian plan seemed to be conceived with a wholesome far-reaching approach including for instance also financial benefits for the implementation of the R&D private sector, although no fundings directly tailored to the digitalization process of this latter sector had been allocated.
Nevertheless, unlike the German strategy, the Italian plan has not been effective as the German one in providing several cross-cutting activities to strength linkages between the public research sector, the private, as well as the policy one, which were already strongly pre-existing in the case of Germany [44], [45]. One possible explanation for this phenomenological observation might find its roots already into the intrinsic design nature of the Italian plan, which has been ideated in a very sector-specific way, without embracing a holistic approach along the products value chain. As a matter of fact, the hyper- and super-amortization incentives were uniquely addressed to the I4.0 development of the production process, without taking into account all the other steps of the value chain which might undergo into digitalization process. Besides, differently from the German strategies, the Italian I4.0 plans have always been focused exclusively on the implementation of the private sector, without perceiving the potential synergistic effect which might be established between the private and public research sectors.
Finally, in support of this latter hypothesis, the Italian plan has indeed been drafted by a single Ministry (Ministry of Economical Development) alone, instead of being the results of joint cross-ministerial efforts, as it was the case for Germany (Development union and Expert commission Research and Innovation) [19], [22]. In addition, unlike the German strategy, the share and human capital together with a program focused on the diffusion of cutting-edge technologies have not been included as part of the plan and supporting programs for the implementation of this latter one neither.
Later on, in 2018 the Italian strategy has been updated with the introduction of further financial benefits for investments addressed to new instrumentations and tools supporting I4.0 transition with a plan titled Piano Nazionale Impresa 4.0 (PNI 4.0) [46]. On energy level, the reduction of the final energy consumption provided by these interventions has been assessed to correspond to 2,8 Mtoe by 2030 [47]. In terms of environmental and energetic benefits, Germany seemed to have been more forefront with respect to Italy, with a well-defined strategy specifically focused on environmental and energetic objectives. Indeed, still in year 2010, an updated version of the “Hightech Strategie” (HTS) has been published with the title of “Hightech Strategie 2020-Ideas. Innovation. Prosperity”, whose main objectives where less centred on specific technologies, rather on concrete solutions to global challenges [19]. To this latter extent, five pillars have been introduced named as “Zukunftsprojekte” (Future projects), such as: climate and energy, health and food, mobility, security and communication, whose implementation would have been feasible only upon adoption of the corresponding “action plan”. This HTS Action plan entered into the public domain two years later, with the designed investment by the German Government of 27 EUR billions for the implementation of the strategic initiatives over 2010-2013 time frame [48]. This updated strategy has constituted the very first scenario for the linkage between digitalization and its corresponding effects on climate and energy level [49], [50], laying also the foundations for the so-called nowadays “digital ecology” [51], [52]. In particular, the strategy aimed at the implementation and intensification of the research sector.
On a later stage, in November 2020 the political manoeuvres of the new Italian plan aiming at implementing the I4.0 revolution went into effect. This latter plan, titled “Piano Nazionale Transizione 4.0” (Transition National Plan 4.0), basically differs from the previous one (PNI 4.0) for the financing system of the incentives, which lasted until 2023, rather than for the contents. Indeed, in the most recent Italian plan the financial benefits consist in tax credits for companies and in this regard the Italian State has allocated European funds corresponding to 13.381 EUR billion, to which are added 5.08 billion of the Complementary Fund (a supplementary fund with national resources) [53]. Unlike the previous Italian plan (PNI 4.0), in the most recent one also financial benefits for training courses and activities have been introduced in order to prepare the human and social capital of companies for the upcoming digital transformation.
Nevertheless, according to the Digital Economic and Society Index report of 2022, Italy outperforms as 25th out of 27 in the “human capital” factor [27]. Concerning the so-called “human capital”, Germany had already moved many steps forward starting from September 2018, when the German Ministry of Education and Research had introduced the latest version of the HTS, titled “High-tech Strategy 2025- Research and Innovation that benefit the people” [23]. This strategy has coined the era of German Industry 5.0, where the human being is at the core of the strategy. One of the core-pillars of the 2025 strategy is represented by the establishment of a strong synergy among industry, science and society. In this regard, the expenditure on Research and Development are planned to be increased to 3.5% of German GDP by 2025. Very recently, in March 2023, the German Federal Ministry for Economy and Climate Protection (Bundesministerium fuer Wirtschaft und Kilmaschutz) has launched a strategy titled “Reaching a Climate Neutral Prosperity” (“Wohlstand klimaneutral erneuern”), which aims at strengthening the industrial competitiveness through decarbonisation [54].
Finally, in December 2020, the Italian Ministry for the technological innovation and digitalization has published an operational plan for the implementation of the National Strategy for Digital Skills [55], which included the education, training and ICT upskilling of the workforce and general population. According to an assessment carried out by the Italian government in 2021 [56], a significant amount of the aforementioned initiatives and activities have been correctly carried out or concluded. At the end of January 2022, the Italian government has also announced the future establishment of a fund (Fondo per la Repubblica Digitale) of 350 million to support initiatives on digital skills and train 2 million citizens in the time frame 2022-2026 [57].
In summary, as evidenced by the comparison among the two analysed policies and regulative frameworks, the strategy design nature would represent one of the main responsible key-factors for the differentiated development among the two countries [58]. In support of this latter hypothesis, the German policy framework, besides being further in time and in number of launched strategies since it invented the system itself, it generally resulted as a joint cross-ministerial efforts which was then further reflected into a drafted strategy with distinctly more multi-sector and holistic nature with respect to the Italian plans. Nevertheless, Italy is proceeding at remarkable pace in the last years in order to fill the gap with frontrunners countries, also on policy level, as demonstrated by “Repubblica Digitale” initiatives.
The digital transformation is at the core of the European Recovery and Resilience Plan, aiming at delivering a comprehensive and sustainable transformation across all sectors of economy, by evening the digitalization stage among European countries. In this regard, the European Parliament together with the EU Commission has introduced on Sept. 2021 the so-called “Path to the Digital Decade”, establishing Digital Targets for 2030, in order to pave a collective progress in shaping the digital transformation of the Member States. Indeed, although the frontrunners countries have remained unchanged during the past five years (such as: Finland, Denmark, Netherland, Sweden and Ireland), a substantial group of country clusters among the European average and is continuously progressing at remarkable pace, being Italy part of this latter group.
The Digital Decade targets established for 2030 might be categorized into four main subgroups: the ICT skills, Digital Transformation of Business, Secure and Sustainable Digital Infrastructure and Digitalization of Public Services [59]. Italy ranks 18th out of 27 in Digital Economic and Society Index (DESI) [27]. In particular, Italy ranks behind the European average on the Human Capital index and the Digital Public Services one, with a position in the standings corresponding to 25th out of 27 and 19th, respectively (Figure 1). Concerning the Human Capital, as evidenced by the DESI report 2022, only 46% of people possess at least basic digital skills compared to the 54% of European average, while the gap becomes smaller for the above basic digital skills, where 23% of Italian people meet the criteria compared to the 26% of the European average. In this regard, Italy is working very hard in order to align its Digital skills with the European benchmark, as demonstrated by the creation and adoption of a National Strategy for Digital Skills [60] and the launch of its corresponding operational plan for the implementation of this latter one [55].
Moreover, on the educational and workforce training front, this latter strategy has created a multitude of initiatives, promoting also cutting-edge digital programs, such as the National Artificial Intelligence (AI) PhD program, which offers more than 200 AI PhD curricula spread around 50 different universities within the Italian territory. Also in the Digital Public Services index the Italian score falls behind the European average, with only 40% of Italian users resorting to public digital services against the 65% of EU average. However, the results do not reflect yet the boost that the Resilience and Recovery Plan is supposed to exert on the digitalization process of public services. While, contrary to the two aforementioned indexes, the Italian scores of the Connectivity index and the Integration of Digital technology one are placed rather high on the DESI standings, outperforming above the European average and ranking as 7th and 8th, respectively (Figure 1).
Overall, in order to even European digital development among the Member States, the reinforcement of digital skills on the Human Capital together with that of Public Services in order to be able to withstand and entail also open innovation activities [61] play a key priority for the proximate Italian political manoeuvres. To this latter extent, it is crucial to preserve the continuity of the initiatives scheduled and launched by the National Strategy for Digital Skills [60] and by the Resilience and Recovery Plan.
Bars plot presenting the total DESI score and its four indexes for Italy and Europe. Data are extracted from Ref [27]
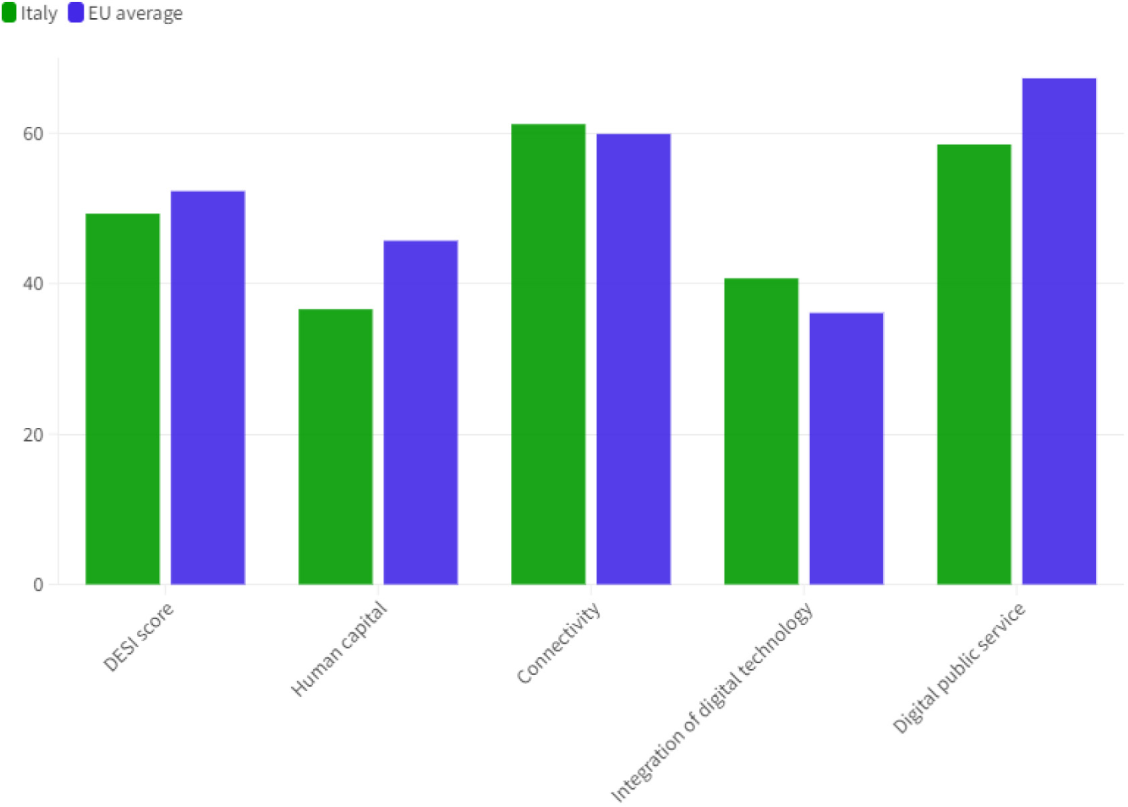
Among the aforementioned DESI indexes, the Integration of Digital Technologies deserves a particular attention while discussing the Italian case. As a matter of fact, Italy is the country of small-medium enterprises (SMEs) (99.3% of the total number of enterprises) [62] and the adoption feasibility of digitalization measures strongly depend also on the enterprise size [63]. On this subject, the so-called Digital Intensity Index (DII) has been designed on purpose to measure the use of different technologies at enterprise level. As expected and also reported by DII for 2021, large companies in EU are more digitalized than SMEs, in particular on cloud service usage, on ERP software package to share information between functional areas, on Customer Relationship Management technology, on buying intermediate-advanced CC services and on social media usage [27]. This could be partially attributable to the fact that SMEs usually don’t have enough manpower to forecast ahead and beyond their own product and production range, hindering in this way new areas and emerging technology discovery.
In addition, several SMEs don’t possess the financial possibility to invest in ground-breaking technologies, consequently losing money by focusing on the wrong ones and they also struggle to digitalize internationally because they were not initially conceived and designed to scale in that way [64]. In this latter regard, open innovation activities could foster the adoption of I4.0 technologies in SMEs through the deployment of technological capabilities [65], [66]. However, due to their flexibility and coherent culture, SMEs posses a high degree of innovation and evolution capacity, which result difficult for large firms to emulate [63], making the unleashing of their digital potential a possible game changer in the industrial sector.
Last but certainly not least, the employment capability of Artificial Intelligence plays a crucial role in the digital transformation of industries. In the most recent years the huge and multitask potential of AI has captured the interest of the major industrial players, who have started to invest significant amounts of money in the implementation of AI-based industry-tailored technologies. Among the most cutting-edge application of AI, its employment in R&D facilities for accelerating material/drug discovery has marked a real breakthrough for the innovative digital industry [30], [31]. Indeed, the society is making huge strides in developing theoretical tools (e.g. Machine learning (ML), within which different essential sub-categories, such as: Artificial Intelligence (AI) and Neuronal Network) which can provide beneficial support to multiple units in industry, not only on the production chain, rather also in other key units across the value chain of a product, such as in research & development. Additionally, the huge potential of machine learning, where it could become possible to couple technical and engineering expertise with mathematical analysis tools, deserves to be included with a central role also into the energy and sustainability level. Indeed, ML and AI tools can support and accelerate the material/compounds discovery and predict their functional performance, by drastically decreasing the amounts of trials needed to achieve an “optimum” in product discovery process and consequently immensely reducing the amount of energy, raw materials and time needed to reach R&D goals.
Nowadays these latter ML and AI applications are still at embryonal stage in the European industry, although in Mittel Europe, which is the cradle of I4.0 revolution, several large companies started to look into this direction for their R&D units. In this regard, the Italian industrial scenario is still behind and several efforts in terms of knowledge deployment of cutting-edge ML and AI applications and workforce education, as well as training are still needed in order to catch up with the frontrunner’s development (Mittel-European countries). The reason of this latter phenomenon might find its roots into the different strategic approach of Germany compared to the Italian one, being the German one more “holistic”-oriented and having been capable to establish several linkages between the public research sector and the private one, where instead the Italian plans seem to have not fully succeed at yet.
An additional explanation certainly lies in the Italian enterprises’ taxonomy. Indeed, as mentioned above, Italy is the country of SMEs [62], while R&D innovation together with cutting-edge digitalization interventions and applications suit better to large companies, due to their intrinsic structural nature. For small-medium enterprises the problem is not limited to the R&D sector, SMEs indeed struggle at European level to meet the Digital Targets fixed by the “Path to the Digital Decade” for 2030 in several “digital indexes”. For instance, one target is constituted by reaching the 75% of European companies dealing with “Big data”, but, as reported in Table 1, in 2020 only the 14% of SMEs used big data, compared to the big companies which possess the lion’s share with 34%. The same for AI technologies employment, where the 7% of SMEs exploit AI technological potential, compared to the 28% of large enterprises [27].
Adoption of key-digital technologies by enterprise size. The listed percentages refer to 2021, with the exception of “Big data”, which refers to 2020. Adapted from Ref. [27]
Digital technology (% enterprises) | SMEs | Big Companies |
---|---|---|
Electronic information sharing | 38% | 81% |
Social media | 28% | 61% |
Big data | 14% | 34% |
Cloud* | 33% | 60% |
AI | 7% | 28% |
SMEs selling online | 18% | 38% |
Selling online cross-border | 9% | 24% |
In summary, European small-medium enterprises still have a long way ahead in order to reach the goals scheduled by the “Path to the Digital Decade”, such as reaching the 90% of basic DII (Digital Intensity Index), where the only exception are constituted by Finland, Denmark, Malta and Sweden. Nevertheless, the Italian small-medium enterprises rank above the European average for possessing at least basic level of digital intensity, for Cloud and e-invoices, as reported by DESI report for 2022 (Table 2). While, they fall slightly behind the European average for social media, big data, AI, electronic sharing information, online (and cross-border) selling and consequently also for e-Commerce turnover, as well as for ICT for environmental sustainability [27].
Comparison between Italy and Europe of Key-parameters for Digital Technology Integration. The listed percentages refer to 2021, with the exception of “Big data” and “e-Invoices”, which refer to 2020. Adapted from Ref. [27]
Parameter | Italy | EU |
---|---|---|
SMEs with at least a basic level of digital intensity (% SMEs) | 60% | 55% |
Electronic information sharing (% enterprises) | 32% | 38% |
Social media (% enterprises) | 27% | 29% |
Big data (% enterprises) | 9% | 14% |
Cloud† (% enterprises) | 52% | 34% |
AI (% enterprises) | 6% | 8% |
ICT for environmental sustainability (% enterprises having medium/high intensity of green action through ICT) | 60% | 66% |
e-invoices (% enterprises) | 95% | 32% |
SMEs selling online (% enterprises) | 13% | 88% |
e-Commerce turnover (% SMEs turnover) | 9% | 12% |
Selling online cross-border (%enterprises) | 7% | 9% |
As evidenced by a survey conducted over a sample of 238 manufacturing companies in Italy, whose main objective was to explore and assess the impact of I4.0 development on energy efficiency, several advantages on the energy and sustainability levels have been provided by the I4.0 performed interventions [67]. In this latter regard, thermal and electrical consumption costs, as well as water and waste management ones have been subjected to analytic evaluation. In Figure 2, the survey results have been reported as percentages calculated either by the number of increased-related answers or by the neutral ones, as well as the decrease-related ones divided by the total number of answers for each parameter. While, the not available answers have been excluded from the calculation. In details, the increase-related answers have ensembled all the positive ranges, such as: 0-1%, 1-5%, 5-10%, 10-20% and >20%. Conversely, the decrease-related answers have ensembled all the negative ranges, which are identical in absolute values to the positive ones. In terms of numerical amount, the total number of responders corresponds to 127, 130, 150 and 151 for waste management, water consumption cost, for the electrical and thermal consumption ones, respectively. The non-available answers is constituted by the differences between the above reported responders’ number and the survey sample (238 responders).
Bars plot presenting the percentages of the surveyed companies which recorded either a cost increase or a cost decrease.. Colour-code: green: cost decrease; grey: neither an increase nor a decrease and orange: cost increase. Data are extracted from Ref [67]
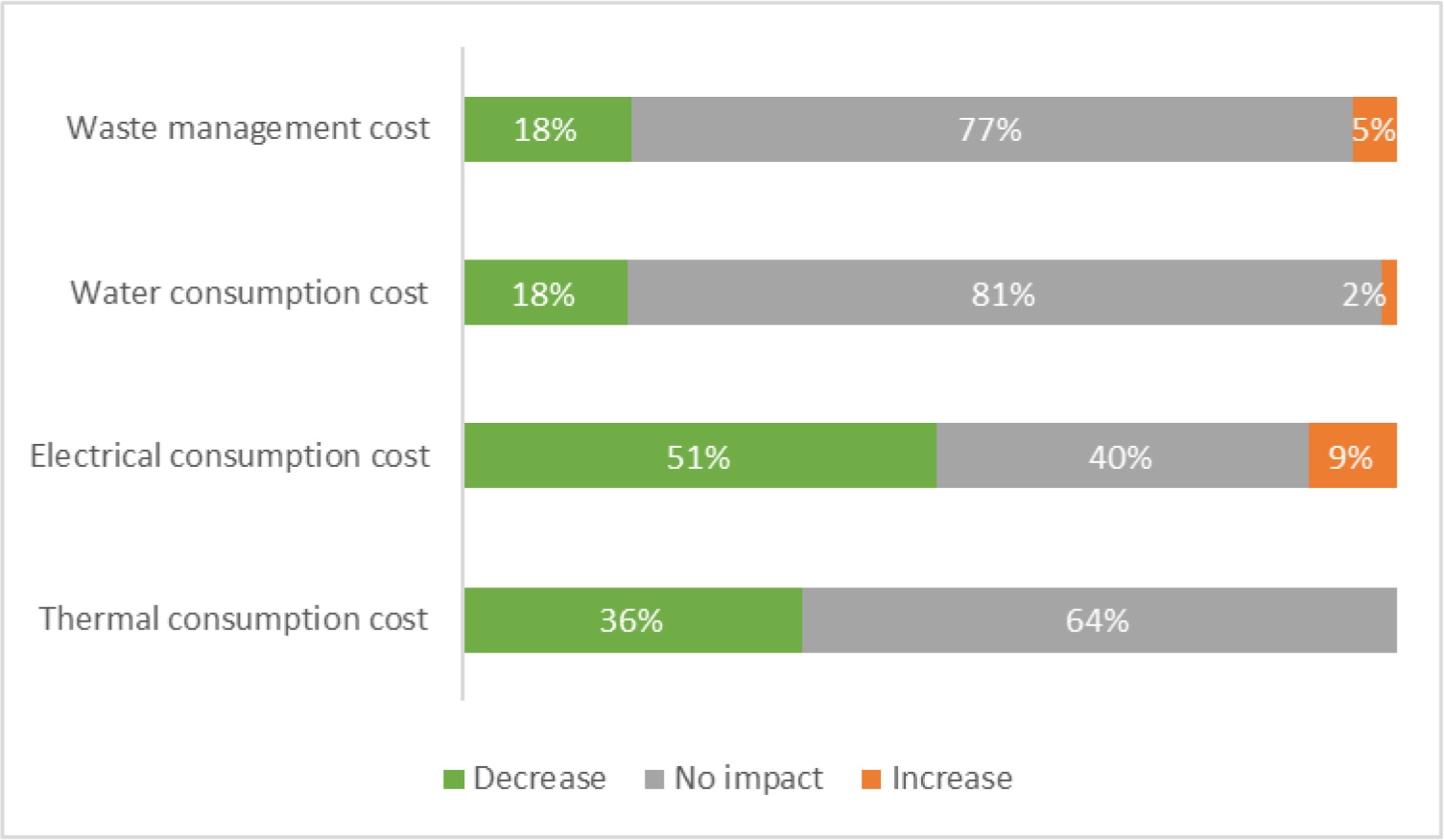
In the case of energetic costs, both the thermal and electrical ones have recorded costs decrease with very significant frequency among the survey companies. In particular, the costs decrease associated to the electrical energy has been reported in a higher sample of surveyed enterprises with respect to that related to the thermal ones. This result might be ascribable to several reasons. First of all, in accordance with the Italian Energy Balance for the year 2020, the electricity is the main energy source consumed by the industrial sector.
Secondly, as reported by “Technical assistance services to assess the energy savings potentials at national and European level” [68], around the 70% of potential energy savings in the industrial sector lies in the thermal energy. Therefore, the found results can be perfectly contextualized within the European trend for the yet unexpressed thermal energy saving potential in industry. Finally, the more frequent decrease of electrical energy consumption with respect to the thermal one might be attributable to the fact that I4.0 interventions deal with instruments/facilities which are alimented by electrical energy in comparison to those which consume the thermal one.
Nevertheless, in the case of electrical energy also a costs increase has been registered for the 9% of the surveyed companies. A possible explanation of this latter phenomenon might lie in the intrinsic nature of the performed I4.0 measures. Indeed, for instance, the automation and process control systems require additional electrical energy demand. Moreover, I4.0 interventions unleash their primary potential into maximising the productivity, which does not always imply an enhancement of energy efficiency.
While, the water and waste management cost have neither registered an increase or a decrease for the majority of the survey companies, being however the decrease related percentage higher in absolute values in comparison with the increase related one.
Overall, the I4.0 carried out interventions have expressed their heuristic potential value, by positively affecting also the energetic and sustainability-related dimensions of manufacturing companies. This phenomenon occurred, although the intrinsic intentions of the Italian Plans lied in the competitiveness enhancement of the national industry, instead of possessing a more holistic approach focused on environmental and sustainability issues like the German strategy already launched in 2015 and the following ones [19], [22], [23], [54].
The aforementioned survey, whose major details are reported in Ref [67], has also investigated the potential obstacles that companies incurred into the I4.0 implementation process together with the parameters which have acted as drivers for these latter interventions, as reported in Figure 3 and Figure 4, respectively. The survey data on I4.0 intervention barriers and drivers have been evaluated and analysed taking into account only the positive answers, such as “does apply completely” and “does rather apply”. Therefore, the shown percentages in Figure 3 and Figure 4 represent the positive answers for a certain barrier or driver normalized over the total number of positive answers along all investigated barriers or drivers. In detail, the possible response choices for the barriers and drivers investigations were the following ones: does apply completely, does rather apply, neutral, does rather not apply, does not apply at all, not available answer.
Pie plot representing the barriers for I4.0 intervention uptake, as evidenced by the survey data and expressed as percentages calculated by the positive answers for a certain barrier normalized over the total number of positive answers along all investigated ones. Total number of responders corresponds to 238. Data are extracted from Ref [67]
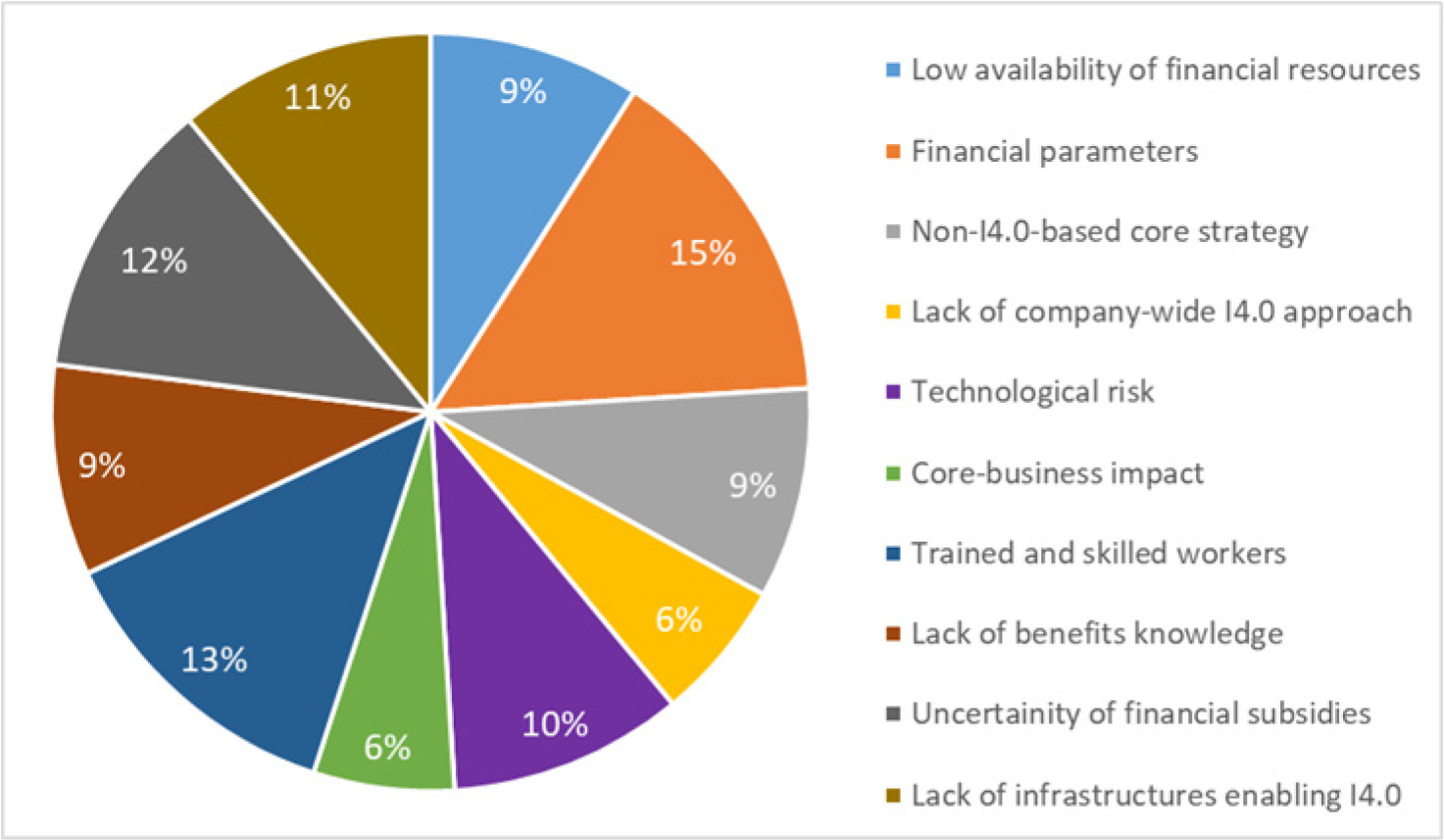
The analysis of the survey data highlights that the major barriers to I4.0 interventions uptake are constituted by financial parameters, such as high investment costs, long payback times and low internal rate returnThe other most frequently reported obstacles are represented by the lack of availability of trained and skilled personnel able to employ I4.0 technologies, the lack of I4.0-based core strategies within the company and that of suitable infrastructure to enable the digital transition. Interestingly, among the barriers, the lack of a company-wide I4.0 approach is represented by the lowest percentage (6%), suggesting either that the digital transformation takes place across all company levels in the majority of the surveyed Italian companies or that these latter ones do not perceive a holistic approach along the entire value chain as a key-factor for a successful core strategy-integrated digital transformation.
Finally, the uncertainty of financial subsidies plays also a significant role among the reported obstacles (12% of the identified barriers survey companies), implying that further efforts from the Italian governments are needed in order to catalyse the uptake of I4.0 interventions within the Italian manufacturing systems.
The production-related parameters, such as optimization and enhanced flexibility of the production process together with a decrease of the production time, possess the lion’s share among the factors which acted as drivers for I4.0 interventions shown in Figure 4. While, the product/service customization can be found at the bottom of the ranking, with the lowest percentage of the survey companies corresponding to 7%. These latter results might be a confirmation that the companies conceive the digitalization and address their digital transformation-related efforts mostly only as an implementation of production step rather than exploring its potential on the final product and thus performing digital interventions along the entire value chain, consequently adopting a holistic transformative digital approach.
Pie plot representing the drivers for I4.0 intervention uptake, as evidenced by the survey data and expressed as percentages calculated by the positive answers for a certain driver normalized over the total number of positive answers along all investigated ones. Total number of responders corresponds to 238. Data are extracted from Ref [67]
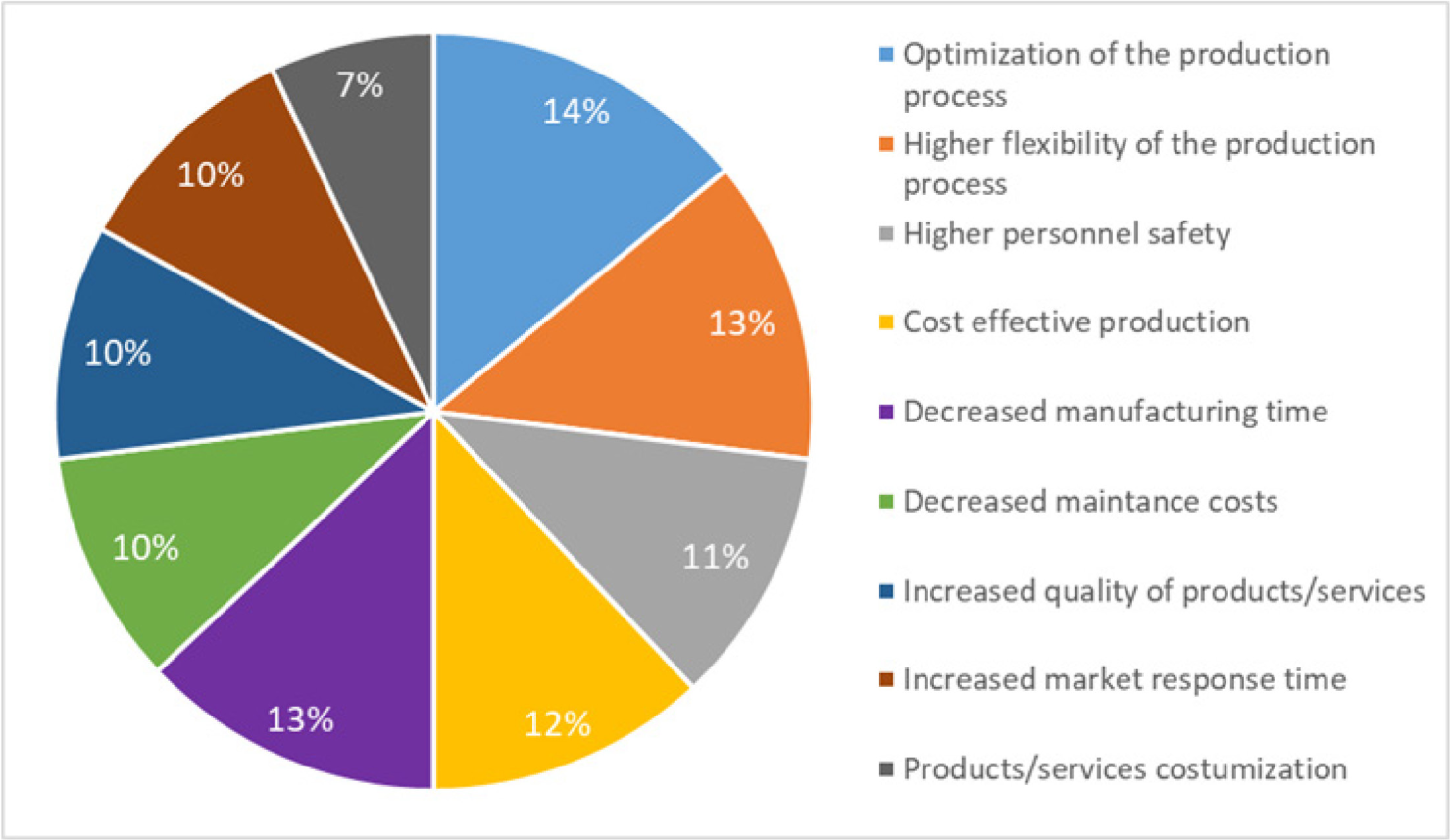
As evidenced by a concept study developed by Bogner et al. on the status quo of the digitalization degree of the manufacturing industry in Germany, it would be an oversimplification to associate the fourth industrial revolution only with logistics as well as production and manufacturing areas [5]. Indeed, starting from the definition of the fourth industrial revolution as systematic increase in the flexibility of products and corresponding processes through automation, Bogner et al. state that, in order to achieve an I4.0 far-reaching change, the digitalization interventions within the manufacturing companies should go beyond the production process, by enabling completely new processes along the entire value chain, from research and development through manufacturing and sales to services concerning the use of a product.
However, in most of the companies the opportunities to integrate additional functional areas of the production process and the vertical value chain into the digital transformation addressed interventions are rarely considered. This phenomenon strongly applies to the Italian system, where, as evidenced also by the analysis of barriers and drivers for I4.0 interventions uptake shown in Figure 3 and Figure 4, respectively, the digitalization interventions are almost uniquely considered suitable and applicable to the manufacturing process for the productivity and cost-efficiency increment, rather than adopting a holistic vision across the entire value chain of a product. Indeed, according to the survey conducted over the Italian manufacturing system by RSE together in collaboration with the University Milano Bicocca [67], the major drivers for I4.0 interventions uptake concern the optimization of the production system, rather than other functional areas (Figure 4).
In support of this latter hypothesis, the low percentage of the lack of a company-wide I4.0 approach among the barriers (Figure 3) might indicate that a holistic approach across the entire value chain is not yet perceived as a key-parameter essential for a far-reaching digital change among the manufacturing companies. Nevertheless, the adoption of a holistic digitalization process in manufacturing industry could constitute the passepartout for achieving a I4.0 breakthrough able to run over also the SMEs, which might highly benefit from the diffusion and deployment of digital measures which go beyond the manufacturing process.
Given the aforementioned motivational elements, this paper further investigates the intrinsic nature of the adopted I4.0 interventions by the Italian manufacturing players and those considered more suitable and consequently more favourably seen by these latter ones, as shown in Figure 5. As for the barriers and drivers for I4.0 measurements implementation reported in Figure 3 and Figure 4, respectively, the survey data on I4.0 intervention uptake, reported in Figure 5 have been evaluated and analysed taking into account only the positive answers, such as “does apply completely” and “does rather apply”. Therefore, the shown percentages represent the positive answers for a certain intervention, normalized over the total number of positive answers along all investigated interventions. In detail, the positive answers are constituted by the ensemble of “Already implemented and furthers are planned” and “Implemented”.
Pie plot representing the I4.0 intervention uptake, as evidenced by the survey data and expressed as percentages calculated by the positive answers for a certain I4.0 intervention. Data are extracted from Ref [67]
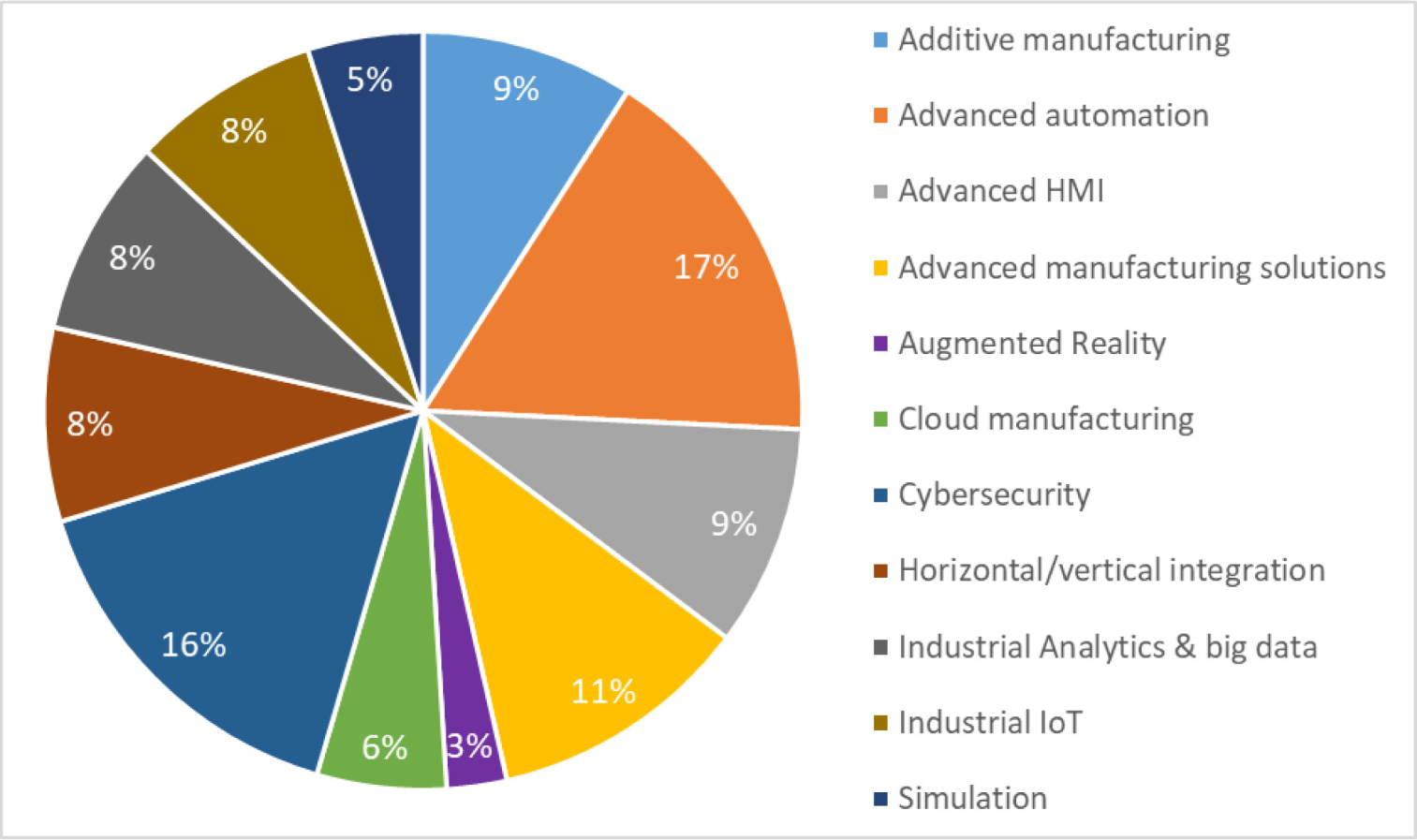
The analysed survey results are in agreement with the expectations, namely that the interventions which are mostly applicable exclusively on the production step of the value chain are those reported with a higher percentage of carried out or planned interventions.
These results can be perfectly contextualized into the taxonomy of the surveyed companies, belonging the most of them to metallurgic sector, followed by non-metallic mineral one. Indeed, unlike the chemical or pharmaceutical sectors for instance, these two sectors possess value chains which are almost exclusively composed by the production step and therefore it is reasonable to find a higher percentage of surveyed companies, which opted for production tailored addressed I4.0 interventions.
In order to deepen the analysis, the herein presented study has further examined the survey data in order to explore and foresee the potential degree of preparation of the Italian manufacturing industry towards a holistic integrated I4.0 wave. To this latter extent, the answers concerning five digital measures, which might be applied also to other value chain steps, have been analysed in detail, as shown in Figure 6. The radar plots shown in Figure 6 are reported as percentage of the shown answer normalized over the total amount of answers concerning the considered intervention (not available answers have been omitted from the calculation).
The selected interventions encompass Advanced Human-Machine Interface (HMI), shown in Figure 6a, which might be applied for instance in the remote-control facilities of R&D units to control the experiments remotely and thus providing several energetic advantages in terms of time and employees transportation. Recent research advances in the field have reported wearable sensor capable to interact in real-time with the surrounding environment, which are generally composed by low dimensional conductive materials (AgNWs and MXenes) combined with stretchable polymers. However, these devices still find several challenges ahead across their scale-up and commercialization process. [69]. Nevertheless, despite the barriers for their up-scaling and roll-out process, flexible noncontact sensing devices embody a huge potential for a large folding fan of sectors, in particular in the medical one [70] Also the Augmented Reality (AR), presented in Figure 6b, might be particularly suitable and useful for other units, beside the productive one. In particular, its high, still at embryonal stage yet, revolutionary potential seems to lie in the health care sector [71], where it could become possible to facilitate and speed up medical diagnosis, like for instance in the case of breast cancer early detection through the combination of AR with depth tri-dimensional scans [72] or in the case of early heart diseases diagnosis, where the heart’s sounds monitoring process can be implemented by coupling with AR, decreasing the possibility of external factors to interfere with the analytical results, such as external noise et cetera [73]. In this context, AR has proven to be a feasible support also in interventional medicine and surgery, as it is the case for interventional radiology [74], or smart glass application for hands-free wound treatment procedure [75], as well as for image-guided surgery [76].
Radar plots presenting the Italian manufacturing surveyed companies propensity towards interventions applicable to different value chain steps. The plots showcase the percentage of responses for the following I4.0 digital technologies: a) Advanced Human-Machine Interface; b) Augmented Reality; c) Industrial Analytics & Big Data; d) Simulation and e) Cybersecurity. Total number of responders corresponds to 238. Data are extracted from Ref [67]
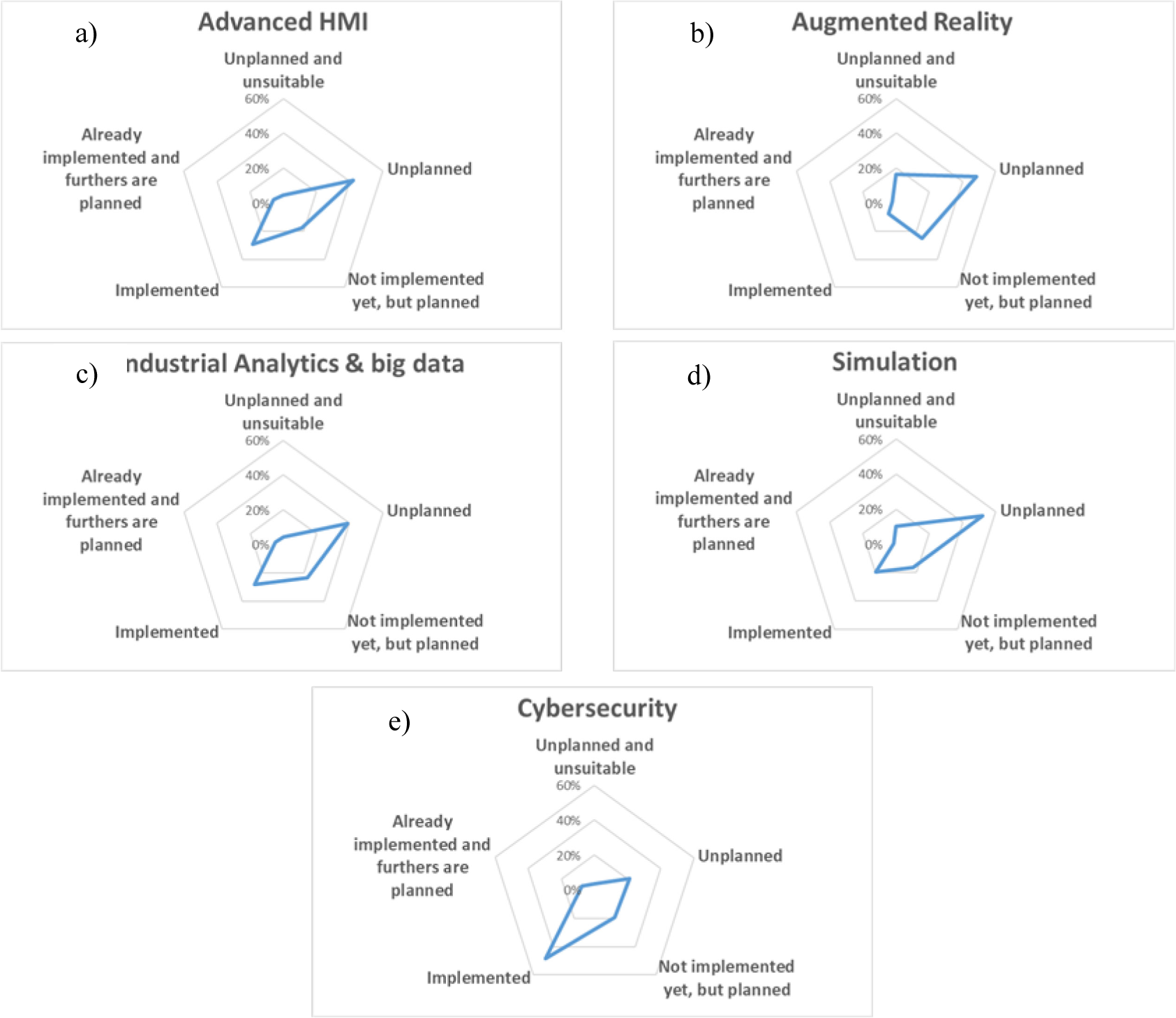
Additionally, AR finds multiple applications also in other different sector, such as in the tourism [77], [78] and sales ones [79], by providing virtual tours and interactive mirrors, respectively. Big-data & analytics together with simulation (Figure 6c and Figure 6d) have the potential lion’s share on R&D units, providing cutting-edge digital tools and solutions for materials/drug discovery and thus drastically decreasing the amount of raw materials, energy and labour costs required by the classical trials-and-error approach, as described above [80].
Finally, also the cybersecurity (Figure 6e) is easily applicable to many different steps of the value chain, perfectly embodying the definition of a multipurpose “holistic-digital-measure”. For all the selected and aforementioned “holistic-digital-measures”, the radar plots shown in Figure 6 highlight that the majority of the survey answers oscillates mostly between two opposites, namely corresponding either to “implemented” or to “unplanned”, being the “unplanned” answer more frequently ticked with respect to the “implemented” one, with the exception of Cybersecurity (Figure 6e), whose implementation rate reaches almost the 60%.
Interestingly, for all the selected measures, the answers “Already implemented and furthers are planned” are 10%, with the exception of AR, which on the other hand shows the highest percentage of “not implemented yet, but planned” and lowest percentage of implementation (Figure 6b). This might suggest that many manufacturing companies are starting to shyly look at this cutting-edge application, although it has rarely been implemented yet.
Overall the analysis of the Italian manufacturing companies approach and propensity towards I4.0 interventions which might be applied to different value chain steps and consequently towards an holistic value chain digital approach has evidenced that the Italian manufacturing industry seems to have acquired use-based knowledge and basic-expertise in the most of the above discussed I4.0 “holistic-digital-measures” and also seems to look with a certain interest towards the less implemented ones, being thus potentially ready to tackle the possibly required upcoming holistic value chain-based digital wave.
Nevertheless, the data suggest also that the Italian manufacturing system is not fully aware yet of the potential of shifting the digitalization objective from the process to the product and thus to exploit the full resources of ICT, as well as digital and automation technologies across the entire value chain. It seems also to lack the perception that this “perspective-change revolution” might be extremely beneficial to the whole industrial national panorama, in particular to SMEs, which might be run over by digital solutions suitable for the development and implementation of their digital transition.
The industry I4.0 has been initially conceived as a tool for strengthening and enhancing the competitiveness of the national industry. Later on, many governments have recognized also the potential environmental beneficial effects of the digitalization measurements and consequently have further promoted the uptake of these latter ones.
Although the driving force for the digital industrial revolution has been basically the same for all countries, it has been developed differently by the national governments, resulting into a diversified digitalization scenario within the European continent. In particular, the digital transformation plays a central role in the European Recovery and Resilience Plan and, in order to reach the European targets framed by the “Path to the Digital Decade” [59], the digitalization development of the Member States has to undergo into an evening process.
In this regard, the Italian case deserves a particular attention and has been the main investigation objective of the herein presented paper, whose analysis has been mainly focused on the digitalization level within the Italian industry. Indeed, although Italy represents the third largest EU economy, it outperforms 18th out of 27th on the Digital Economic and Society Index [27]. In particular, Italy ranks behind the European average on the Human Capital index and the Digital Public Services one, with a position in the standings corresponding to 25th out of 27 and 19th, respectively. While, unlike to the two aforementioned indexes, the Italian scores of the Connectivity and the Integration of Digital technology indexes are placed rather high on the DESI standings, outperforming above the European average and ranking as 7th and 8th, respectively. Moreover, the Integration of Digital Technologies index deserves a particular attention while discussing the Italian case. This latter one has been designed on purpose to measure the use of different technologies at enterprise level and large companies in EU are more digitalized than SMEs, in particular on cloud service usage, on ERP software package to share information between functional areas, on Customer Relationship Management technology, on buying intermediate-advanced CC services and on social media usage. As a matter of fact, Italy is the country of small-medium enterprises (SMEs) (99.3% of the total number of enterprises) [62] and SMEs generally struggle at European level to meet the Digital Targets fixed by the “Path to the Digital Decade” for 2030 in several “digital indexes”.
Indeed, European small-medium enterprises still have a long way ahead in order to fulfil the criteria framed by the “Path to the Digital Decade”, such as reaching the 90% of basic DII (Digital Intensity Index). Nonetheless, the Italian small-medium enterprises rank above the European average for Cloud and e-invoices, as reported by DESI report for 2022 [27]. While, for social media, big data, AI, electronic sharing information, online (and cross-border) selling and consequently also for e-Commerce turnover, as well as for ICT for environmental sustainability Italian SMEs scores fall behind the European average [27].
In order to deepen the analytical investigation over the carried out I4.0 interventions within the Italian industry, the data of a survey previously conducted by our research group have been further analysed [67]. The analytical investigation has identified the lack of a digital holistic-oriented approach as one of the key-parameter responsible for the differentiated industrial digital development between Italy and Germany, which acted as the cradle of I4.0. In support of this latter hypothesis, the survey results have evidenced that the majority of the performed I4.0 interventions can be mainly applied to the production process, rather than to other crucial steps of the value chain. To this latter extent, the survey data analysis suggest that a shift of the I4.0 objective from the production step to the product itself is not yet perceived by the Italian industrial mindset as a passepartout to reach a breakthrough able to boost the Italian digitalization transformative process and to even this latter one to the development stage and pace of the EU frontrunner countries. Conversely to what might be generally perceived at company level, the aforementioned shift of “digital paradigm” could be very beneficial to the whole Italian industrial scenario, in particular to SMEs, which might be run over by digital tools particularly suitable for the development and implementation of their digital transition. Moreover, unlike Italy, on the policy level Germany seemed to have a way more holistic-oriented legislative approach towards the industrial digitalization. This latter policy framework has then been reflected into the I4.0 implementation process and might constitute a key-parameter for the differentiated development among the front runners and the other EUcountries.
Finally, as discussed above in detail in the “Italian I4.0 scenario on policy level and cross-comparison with the German system” chapter, although the Italian government seems to be very keen on paving the way for a holistic digital transformation, a fast boost on the policy level based on setting higher ambitions and objectives, as well as a more strategic regulative framework and on supporting research and education is needed in order to bridge the gap with the digitalization industrial level of the EU frontrunners countries.
This work was financed by the Research Fund for the Italian Electrical System in compliance with the Decree of Minister of Economic Development on 16 April 2018.
The authors also acknowledge the CESISP of the Milano Bicocca University for the support in survey development, deployment and corresponding data analysis.
AI | Artificial Intelligence |
AR | Augmented Reality |
CC | Cloud Computing |
DESI | Digital Economic and Society Index |
DII | Digital Intensity Index |
ERP | Enterprise Resource Planning |
GDP | Gross Domestic Product |
HMI | Human-Machine-Interface |
HTS | High-Tech Strategie |
ICT | Information and Communication Technology |
I4.0 | Industry 4.0 |
ML | Machine Learning |
PNI4.0 | Piano Nazionale Impresa 4.0 |
R&D | Research and Development |
SME | Small-Medium Enterprise |
- Securing the Future of German Manufacturing Industry: Recommendations for implementing the strategic initiative INDUSTRIE 4.0, Final Report of the Industrie 4.0 Working Group, 20132024, https://www.din.de/resource/blob/76902/e8cac883f42bf28536e7e8165993f1fd/recommendations-for-implementing-industry-4-0-data.pdf
- , , 2015
Agility and Industry 4.0 Implementation Strategy in a Quebec Manufacturing SME ,Sustainability , Vol. 14 (13), 2022, https://doi.org/https://doi.org/10.3390/su14137884
, Cluster role in industry 4.0 – a pilot study from Germany ,Competitiveness Review: An International Business Journal , Vol. 31 (1),pp 54–82 , 2021, https://doi.org/https://doi.org/10.1109/GIOTS.2019.876641410.1108/CR-10-2019-0091
, Study Based Analysis on the Current Digitalization Degree in the Manufacturing Industry in Germany ,Procedia CIRP , Vol. 57 ,pp 14–19 , 2016, https://doi.org/https://doi.org/10.1016/j.procir.2016.11.004
, - , , Advances in Human Factors and Systems Interaction, 2020
- Holistic Digitalization: Strategy, Transformation, and Implementation., 20212024, https://www.researchgate.net/publication/356695507.
The Art Of Holistic Digitalisation: A Meta-View On Strategy, Transformation, Implementation, And Maturity ,International Journal of Innovation Management , Vol. 26 (03),pp 2240007 , 2022, https://doi.org/https://doi.org/10.1142/S1363919622400072
, Digitalization in the financial industry: A contingency approach of entrepreneurial orientation and strategic vision on digitalization ,European Management Journal , Vol. 39 (3),pp 317–326 , 2021, https://doi.org/https://doi.org/10.1016/j.emj.2020.04.008
, Lean 4.0: A new holistic approach for the integration of lean manufacturing tools and digital technologies ,International Journal of Mathematical, Engineering and Management Sciences , Vol. 5 (5),pp 854–868 , 2020, https://doi.org/https://doi.org/10.33889/IJMEMS.2020.5.5.066
, - , Information Technology as the Basis for Transformation into a Digital Society and Industry 5.0, (IT&QM&IS), 2019
Upgrading strategies for the digital economy ,Global Strategy Journal , Vol. 11 (1),pp 34–57 , 2021, https://doi.org/https://doi.org/10.1002/gsj.1364
, - , , Shaping the Digital Enterprise: Trends and Use Cases in Digital Innovation and Transformation, 2017
Corporate Restructuring and New Forms of Organizing: Evidence from Europe ,MIR: Management International Review , Vol. 39 (2),pp 41–64 , 1999, https://doi.org/https://doi.org/10.1007/978-3-322-90991-6_4
, Regulations and Technology Diffusion: Cross Subsidies and Digital Infrastructure Creation ,Annals of Public and Cooperative Economics , Vol. 84 (2),pp 159–178 , 2013, https://doi.org/https://doi.org/10.1111/apce.12011
, - , , 2013
Big Data Processing for Renewable Energy Telemetry Using a Decentralized Cloud M2M System ,Wirel Pers Commun , Vol. 87 (3),pp 1113–1128 , 2016, https://doi.org/https://doi.org/10.1007/s11277-015-2527-7
, Cyber–Physical Production Systems for Data-Driven, Decentralized, and Secure Manufacturing—A Perspective ,Engineering , Vol. 7 (9),pp 1212–1223 , 2021, https://doi.org/https://doi.org/10.1016/j.eng.2021.04.021
, - , Ideas, innovation, prosperity–high-tech strategy 2020 for Germany., Federal Ministry of Education and Research Bonn, 2010
BMBF: Die Hightech-Strategie für Deutschland ,Datenschutz und Datensicherheit - DuD , Vol. 30 (10),pp 665–666 , 2006, https://doi.org/https://doi.org/10.1007/s11623-006-0175-5
, - What can policymakers learn from Germany’s Industrie 4.0 development strategy?, 201820242024, https://downloads.unido.org/ot/11/71/11712839/WP_22.pdf.
- IKT-Strategie der Bundesregierung ‘Deutschland Digital 2015,, 20102024, https://www.post-und-telekommunikation.de/PuT/1Fundus/Dokumente/5._Nationaler_IT-Gipfel_2010_Dresden/ikt-strategie-der-bundesregierung,property=pdf,bereich=bmwi,sprache=de,rwb=true.pdf.
- , First Mission Analysis Report of the Scientific Support Action to the German Hightech Strategy 2025 — Setting the stage: Positioning the missions in the socio-technical system., 2020
Industry 5.0—A Human-Centric Solution ,Sustainability , Vol. 11 (16), 2019, https://doi.org/https://doi.org/10.3390/su11164371
, - Industry 5.0 and the future of work: making Europe the centre of gravity for future good-quality jobs, 20232024, https://op.europa.eu/en/publication-detail/-/publication/948cbd47-2147-11ee-94cb-01aa75ed71a1/language-en/format-PDF/source-289416748.
Society 5.0-its historical logic and its structural development ,Journal of Scientific Reports , Vol. 2 (1),pp 32–42 , 2020, https://doi.org/https://doi.org/10.5281/zenodo.3731954
, - , Digital Economic and Society Index (DESI) 2022.
Defining, conceptualising and measuring the digital economy ,Development Informatics working paper , (68), 2017, https://doi.org/https://doi.org/10.2139/ssrn.3431732
, Digital inequalities and why they matter ,Inf Commun Soc , Vol. 18 (5),pp 569–582 , 2015, https://doi.org/https://doi.org/10.1080/1369118X.2015.1012532
, Artificial intelligence for materials discovery ,MRS Bull , Vol. 44 (7),pp 538–544 , 2019, https://doi.org/https://doi.org/10.1557/mrs.2019.158
, Machine learning-driven new material discovery ,Nanoscale Adv. , Vol. 2 (8),pp 3115–3130 , 2020, https://doi.org/https://doi.org/10.1039/D0NA00388C
, Artificial intelligence and machine learning in design of mechanical materials ,Mater. Horiz. , Vol. 8 (4),pp 1153–1172 , 2021, https://doi.org/https://doi.org/10.1039/D0MH01451F
, Accelerating the discovery of materials for clean energy in the era of smart automation ,Nat Rev Mater , Vol. 3 (5),pp 5–20 , 2018, https://doi.org/https://doi.org/10.1038/s41578-018-0005-z
, Accelerated discovery of CO2 electrocatalysts using active machine learning ,Nature , Vol. 581 (7807),pp 178–183 , 2020, https://doi.org/https://doi.org/10.1038/s41586-020-2242-8
, Toward Excellence of Electrocatalyst Design by Emerging Descriptor-Oriented Machine Learning ,Adv Funct Mater , Vol. 32 (17),pp 2110748 , 2022, https://doi.org/https://doi.org/10.1002/adfm.202110748
, Discovery and design of soft polymeric bio-inspired materials with multiscale simulations and artificial intelligence ,J. Mater. Chem. B , Vol. 8 (31),pp 6562–6587 , 2020, https://doi.org/https://doi.org/10.1039/D0TB00896F
, An artificial intelligence-aided virtual screening recipe for two-dimensional materials discovery ,NPJ Comput Mater , Vol. 6 (1),pp 106 , 2020, https://doi.org/https://doi.org/10.1038/s41524-020-00375-7
, - , , 2020
- AI on drugs: Can artificial intelligence accelerate drug development? Evidence from a large-scale examination of bio-pharma firms, 2021, http://dx.doi.org/10.2139/ssrn.3524985.
Can artificial intelligence accelerate preclinical drug discovery and precision medicine? ,Expert Opin Drug Discov , Vol. 17 (7),pp 661–665 , 2022, https://doi.org/https://doi.org/10.1080/17460441.2022.2090540
, A Deep Learning Approach to Antibiotic Discovery ,Cell , Vol. 180 (4),pp 688-702.e13 , 2020, https://doi.org/https://doi.org/10.1016/j.cell.2020.01.021
, Use of Artificial Intelligence in the Design of Small Peptide Antibiotics Effective against a Broad Spectrum of Highly Antibiotic-Resistant Superbugs ,ACS Chem Biol , Vol. 4 (1),pp 65–74 , 2009, https://doi.org/https://doi.org/10.1021/cb800240j
, - , Piano Nazionale Industria 4.0, Italian Trade Agency, 2017
Knowledge and Competitive Advantage in the Synthetic Dye Industry, 1850–1914: The Coevolution of Firms, Technology, and National Institutions in Great Britain, Germany, and the United States ,Enterp Soc , Vol. 1 (4),pp 699–704 , 2000, https://doi.org/https://doi.org/10.1093/es/1.4.699
, The determinants of national innovative capacity ,Res Policy , Vol. 31 (6),pp 899–933 , 2002, https://doi.org/https://doi.org/10.1016/S0048-7333(01)00152-4
, - , Piano Nazionale Impresa 4.0, 2018
- Economico, “National Integrated Plan For Energy And Climate”, (In Italian, Piano Nazionale Integrato Per L’energia E Il Clima, 20192024, https://www.mimit.gov.it/images/stories/documenti/PNIEC_finale_17012020.pdf.
- Federal report research and innovation 2012”, (In German Bundesbericht Forschung und Innovation 2012”), Bundesministerium für Bildung und Forschung Bonn, 20122024, https://www.bundesbericht-forschung-innovation.de/files/2012%20BuFI.pdf.
Unleashing the convergence amid digitalization and sustainability towards pursuing the Sustainable Development Goals (SDGs): A holistic review ,J Clean Prod , Vol. 280 ,pp 122204 , 2021, https://doi.org/https://doi.org/10.1016/j.jclepro.2020.122204
, - , , Proceedings of Topical Issues in International Political Geography, 2023
- Federal Ministry for the Enviroment, Umweltgerechte Digitalisierung., 2024, https://www.bmuv.de/umweltpolitische-digitalagenda/umweltgerechte-digitalisierung.
- , Digital Product Passport Policy in Europe: Potential Shift towards Circular Product Strategies, KU Leuven, Leuven, 2023
- , Corporate renewal through the “National Transition Plan 4.0.”: A look at the tax credit in Research, Development, Innovation and Design and a comparison with the German system”, (In Italian, “Rinnovamento aziendale tramite il “Piano Nazionale Transizione 4.0.”: Uno sguardo al Credito d’imposta in Ricerca, Sviluppo, Innovazione e Design ed una comparazione con l’ordinamento tedesco”), University Ca’ Foscari Venezia, Venezia, Italy, 2021
- , Transformation to a climate-neutral industry: Green lead markets and climate protection agreements. Report from the Scientific Advisory Board at the Federal Ministry for Economic Affairs and Climate Protection (BMWK)”, (In German, Transformation zu einer klimaneutralen Industrie: Grüne Leitmätkte und Klimaschutzverträge. Gutachten des Wissenschaftlichen Beirats beim Bundesministerium für Wirtschaft und Klimaschutz (BMWK)”), Universität zu Köln, 2022
- , National Strategy for Digital Skills. The Operational Plan
- , National Strategy for Digital Skills. The Monitoring Reports of the Operational Plan
- , The Digital Republic Fund is born
- , Promoting innovation in developing countries: a conceptual framework, World Bank Publications, 35542005
- Path to the digital decade programme., 2024, https://www.europarl.europa.eu/RegData/etudes/BRIE/2022/733519/EPRS_BRI(2022)733519_EN.pdf.
- , National Strategy for Digital Skills.
Digital manufacturing challenges through open innovation perspective ,Procedia Manuf , Vol. 42 ,pp 165–172 , 2020, https://doi.org/https://doi.org/10.1016/j.promfg.2020.02.066
, - Eurostat Database., 2024, https://ec.europa.eu/eurostat/web/structural-business-statistics/visualisations.
Antecedents, consequences, and challenges of small and medium-sized enterprise digitalization ,J Bus Res , Vol. 112 ,pp 119–127 , 2020, https://doi.org/https://doi.org/10.1016/j.jbusres.2020.03.004
, Internationalization, digitalization, and sustainability: Are SMEs ready? A survey on synergies and substituting effects among growth paths ,Technol Forecast Soc Change , Vol. 166 ,pp 120650 , 2021, https://doi.org/https://doi.org/10.1016/j.techfore.2021.120650
, Developing technological capabilities for Industry 4.0 adoption: An analysis of the role of inbound open innovation in small and medium-sized enterprises ,Creativity and Innovation Management , Vol. 32 (2),pp 249–265 , 2023, https://doi.org/https://doi.org/10.1111/caim.12551
, How can open innovation support SMEs in the adoption of I4.0 technologies? An empirical analysis ,R&D Management , Vol. 52 (4),pp 615–632 , 2022, https://doi.org/https://doi.org/10.1111/radm.12507
, Energy efficiency in Industry 4.0: Assessing the potential of Industry 4.0 to achieve 2030 decarbonization targets ,International Journal of Energy Production and Management , Vol. 6 (4),pp 371–381 , 2021, https://doi.org/https://doi.org/10.2495/EQ-V6-N4-371-381
, - , Technical assistance services to assess the energy savings potentials at national and European level – Summary of EU results, 2021
Wearable Sensors-Enabled Human–Machine Interaction Systems: From Design to Application ,Adv Funct Mater , Vol. 31 (11),pp 2008936 , 2021, https://doi.org/https://doi.org/10.1002/adfm.202008936
, Flexible Noncontact Sensing for Human–Machine Interaction ,Advanced Materials , Vol. 33 (16),pp 2100218 , 2021, https://doi.org/https://doi.org/10.1002/adma.202100218
, - , , Biomedical Information Technology (Second Edition), 2020
Augmented Reality: Advances in Diagnostic Imaging ,Multimodal Technologies and Interaction , Vol. 1 (4), 2017, https://doi.org/https://doi.org/10.3390/mti1040029
, An augmented reality-supported mobile application for diagnosis of heart diseases ,J Supercomput , Vol. 76 (2),pp 1242–1267 , 2020, https://doi.org/https://doi.org/10.1007/s11227-018-2483-6
, Augmented and Mixed Reality: Technologies for Enhancing the Future of IR ,Journal of Vascular and Interventional Radiology , Vol. 31 (7),pp 1074–1082 , 2020, https://doi.org/https://doi.org/10.1016/j.jvir.2019.09.020
, Digital Transformation in Health Care: Augmented Reality for Hands-Free Service Innovation ,Information Systems Frontiers , Vol. 22 (6),pp 1419–1431 , 2020, https://doi.org/https://doi.org/10.1007/s10796-019-09937-7
, - , Augmented Reality in Image-Guided Surgery, 2017
New realities: a systematic literature review on virtual reality and augmented reality in tourism research ,Current Issues in Tourism , Vol. 22 (17),pp 2056–2081 , 2019, https://doi.org/https://doi.org/10.1080/13683500.2017.1417359
, Exploring the value of augmented reality for tourism ,Tour Manag Perspect , Vol. 35 ,pp 100672 , 2020, https://doi.org/https://doi.org/10.1016/j.tmp.2020.100672
, Augmented reality in retailing: a review of features, applications and value ,International Journal of Retail & Distribution Management , Vol. 47 (11),pp 1125–1140 , 2019, https://doi.org/https://doi.org/10.1108/IJRDM-12-2018-0263
, Digital innovation and the effects of artificial intelligence on firms’ research and development – Automation or augmentation, exploration or exploitation? ,Technol Forecast Soc Change , Vol. 179 ,pp 121636 , 2022, https://doi.org/https://doi.org/10.1016/j.techfore.2022.121636
,